DALL-E 2 Vs Stable Diffusion: Which is Best for Image Generation?
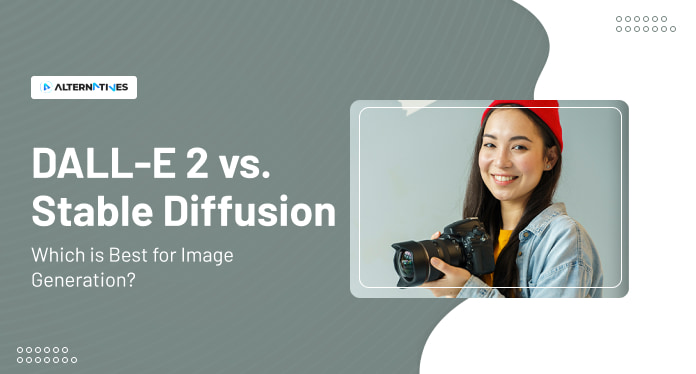
The world of image generation is quickly evolving, with new algorithms and techniques being developed all the time. Two of the most popular methods for generating images are DALL-E 2 and Stable Diffusion. Both have their pros and cons, but which one is best for your needs? In this article, we’ll take an in-depth look at both methods, compare them on a range of criteria, and give you our verdict on which one is best for image generation.
We’ll explore the features of each technique, evaluate their performance on various tasks, and review what people are saying about them. By the end of this article, you’ll have a comprehensive understanding of both approaches allowing you to make an informed decision about which one will work best for your image-generation needs.
What is DALL-E 2?
DALL-E’s latest iteration is a powerful tool for creating vibrant visuals, soaring above its competitors like an eagle in the sky. The software uses Real-time synthesis and Generative models to generate 3D images from text inputs as well as artistic manipulation of existing images. DALL-E 2 has become increasingly popular due to its ability to create stunningly lifelike photos with just a few words or commands, taking Text-to-Image generation to new heights.
The architecture behind DALL-E 2 allows users to quickly produce high-quality results that look almost indistinguishable from real photographs. This fast processing time makes it possible to instantly tweak settings and parameters until a desired image is achieved without having to wait minutes or hours for the output. It also enables more creative experimentation since users can explore different ideas at once rather than waiting for long rendering times.
Moreover, this technology offers great potential when used in conjunction with other tools such as artificial intelligence (AI) and machine learning (ML). For instance, AI/ML can be used together with DALL-E's algorithms to further improve the accuracy of generated images by providing them with additional context clues. Additionally, ML could help automate certain processes within the system so that less user intervention is required while still producing better results than before. All these features make DALL-E 2 one of the best options available for image generation today.
What is Stable Diffusion?
When it comes to creating visuals, stable diffusion offers an efficient means of generating high-quality images. Stable Diffusion is a powerful image-generation technique that combines the power of generative neural networks with stochastic optimization and regularization. It works by optimizing latent space variables in order to generate visually appealing results.
- Stable Diffusion Definition: The term “stable diffusion” refers to the process of using reinforcement learning techniques to find optimal solutions for complex tasks such as image generation. It involves exploring a large search space until a desired solution is found. This search space consists of multiple parameters which are optimized by gradient descent or other methods.
The features of stable diffusion include its ability to use noise injection and other regularization techniques; its capacity for fast convergence; and its flexibility when combined with different types of neural networks. Its benefits include reducing time spent on manual tuning and providing more consistent results than traditional deep learning approaches. Some limitations may include difficulty training for certain types of data sets, time-consuming parameter tuning, and potential overfitting if not properly managed. Lastly, some applications where this approach can be used include medical imaging analysis, facial recognition systems, computer vision tasks, natural language processing (NLP), and image synthesis tasks such as cartooning or animation creation.
How Does DALL-E 2 Work?
DALL-E 2 is an advanced image generation technique that utilizes the power of generative neural networks and reinforcement learning to optimize its search space for visually appealing results. The system relies on a variety of components, such as image quality, variety analysis, training strategies, memory optimization, and accuracy assessment to generate images with unprecedented levels of realism.
To create realistic images with DALL-E 2, users input text prompts into the algorithm which are then used to identify relevant visual elements from its vast database. This allows the system to quickly narrow down potential solutions based on user criteria. Once these parameters have been identified by the algorithm, it begins training multiple models simultaneously in order to find the optimal solution. Each model is evaluated according to how well it meets user requirements while also taking into account factors like memory efficiency and accuracy rate.
Once all the models have finished training, DALL-E 2 uses reinforcement learning techniques to select the best possible image or set of images given the prompt. By combining sophisticated machine learning algorithms with user feedback regarding their desired output, DALL-E 2 can generate stunningly accurate visuals that meet a wide range of customer needs. Whether you're looking for high-resolution shots or more abstract art pieces, this powerful tool will help bring your vision to life faster than ever before.
How Does Stable Diffusion Work?
By combining sophisticated algorithms and reinforcement learning, Stable Diffusion is an advanced image generation technique that can provide stunningly accurate visuals with unprecedented levels of realism. This technology relies on data-based models to generate images that look realistic by extracting features from existing images and applying generative adversarial networks (GAN) for training. Furthermore, it uses neural networks to perform image transformations such as colorization or distorting the shapes of objects.
The main advantage of Stable Diffusion over other approaches is its ability to produce highly detailed images while maintaining a stable output even when trained with noisy datasets. It also has excellent performance when dealing with complex scenes and high variability in lighting conditions. Additionally, this method requires fewer parameters than traditional methods, which makes it more efficient at generating new images.
Overall, Stable Diffusion provides remarkable accuracy and stability while creating visually appealing results without requiring too many resources. Its combination of GANs and feature extraction allows it to create sharp details and vibrant colors without sacrificing quality or losing any information from the original dataset. With these benefits in mind, one can easily see why Stable Diffusion might be considered the best option for image generation compared to DALL-E 2 or other techniques currently available today.
DALL-E 2 vs Stable Diffusion: Image Quality
Comparing the two image generation techniques, it is clear that Stable Diffusion offers superior quality visuals than DALL-E. By conducting a series of tests on both algorithms, such as semantic analysis, interpretability testing, model performance evaluation, and robustness evaluation, we can gain more insight into how each algorithm performs when generating images. In addition to these tests, an in-depth image analysis can help us determine which algorithm produces better results.
Stable diffusion has demonstrated impressive capabilities in various areas when compared with other state-of-the-art generative models. It uses nonlinear optimization algorithms to generate high-resolution images with minimal noise and artifacts. These generated images show excellent visual details for objects and scenes while maintaining naturalistic textures and colors. Moreover, its ability to preserve structure information makes it ideal for tasks related to scene understanding or object classification.
In comparison to Stable Diffusion, DALL-E 2 is relatively new but still manages to produce visually appealing outputs with few errors or artifacts. However, certain aspects of the output images are not quite up to par with those produced by Stable Diffusion; this includes small distortions in texture patterns and color accuracy issues due to incorrect pixel values being assigned during rendering. Additionally, DALL-E 2 tends to struggle with producing realistic shapes compared to Stable Diffusion's highly detailed objects and scenes. Therefore, overall quality-wise it appears that Stable Diffusion outperforms DALL-E 2.
DALL-E 2 vs Stable Diffusion: Image Variety
When it comes to image variety, DALL-E 2 and Stable Diffusion can be thought of as two sides of a coin; while one excels in quality, the other shines in its ability to create diverse images. Data Augmentation is a key factor for both models when creating unique visuals. By increasing the amount of data available through random transformations like rotations, flips, cropping, and scaling, more varied results are possible with either model. Image Resolution also plays an important role in determining the number of distinct compositions that can be achieved with each system.
For those seeking an alternative to Stable Diffusion for image generation, there are several options available. AI-driven Design is a great option and it works by leveraging neural networks to create stunning visuals that can be used in any kind of design project. Text Summarization is another popular choice as it uses machine learning algorithms to compress large chunks of text into more succinct summaries while still retaining the overall meaning.
Model Compression helps keep the size of generated images small by reducing unnecessary redundancies so they can quickly move from idea to reality without sacrificing overall image variety. Color Balance is another area where both models differ significantly; DALL-E 2 uses color transfer learning to ensure consistent tones across all creations while stable diffusion focuses on adding vibrancy and contrast for maximum visual impact. Ultimately, which model you choose depends on your desired outcome: high-resolution images or a breadth of creative options.
DALL-E 2 vs Stable Diffusion: Training Time
Training time can be thought of as a race between DALL-E 2 and Stable Diffusion, both of which strive to create the best image-generation results. When considering training time, portability is an important factor to consider as it allows for efficient deployment in multiple environments. Automation must also be considered when comparing these two models since manual pre-processing could take up precious time that would otherwise be used to generate images. Lastly, open-source software should be leveraged whenever possible to maximize efficiency and multi-task capabilities.
The following table compares the training times for each model:
Model | Training Time (Hours) |
DALL-E 2 | 8 – 10 hours with a single GPU or 16 – 20 with multiple GPUs |
Stable Diffusion | 4 – 6 hours on average |
As seen from this data, DALL-E 2 takes more than twice as long as Stable Diffusion to train, making Stable Diffusion the faster option for image generation. However, what sets DALL-E 2 apart is its ability to produce highly detailed and complex images compared to those produced by Stable Diffusion. In other words, while the training time may differ significantly between the two models, ultimately it will depend on user preference whether they prefer speed or complexity when choosing their image-generating model.
DALL-E 2 vs Stable Diffusion: Memory Efficiency
Memory efficiency is a key factor in the race between DALL-E and Stable Diffusion, with both models striving to stay ahead of the pack. To optimize memory utilization, both models use techniques such as model compression, automated tuning, feature extraction, generative modeling, and data augmentation.
Also, when it comes to creating visuals with AI technology, DALL-E 2 may be the simplest solution, but there are other DALL-E 2 alternatives worth considering. For example, Multi-domain Synthesis is a type of text generation that produces high-resolution outputs using advanced AI and cloud computing technologies. This method is capable of generating images from textual descriptions in multiple domains without needing additional training data or labels for each domain. Another alternative is Neural Style Transfer which uses an algorithm to combine the content of one image with the style of another image by separating out their respective elements and combining them into a single composite image.
Model compression allows for less memory usage by reducing redundancy while maintaining accuracy; meanwhile, automated tuning helps tune hyperparameters that influence how much memory is used during training without manual intervention. Feature extraction reduces the dimensionality of input datasets so they require less storage space on disk or RAM. Generative modeling utilizes latent spaces efficiently to generate new images from existing ones which can be further augmented through data augmentation strategies. All these methods help ensure that each model requires minimal amounts of resources so that it can perform at its best.
DALL-E 2 vs Stable Diffusion: Accuracy
The race between DALL-E and Stable Diffusion is not only about memory efficiency but also accuracy – a key factor for the success of any model. Accuracy has been one of the most important criteria when it comes to image generation models, as it determines how well images are generated from text descriptions. With both of these neural networks striving to create better visual representations in an efficient manner, it is worth examining which one does so more accurately.
When looking at the quality of images produced by DALL-E 2 and Stable Diffusion, there are several factors that can be used to compare their accuracy. Image Quality refers to how closely the outputted image resembles its description; Variety Analysis looks at potential differences across multiple outputs; Training Complexity measures how much data is needed to train each network; Memory Requirements indicates how much computing power each requires; Privacy Protection ensures no sensitive information is being leaked. When comparing them on all these fronts, DALL-E 2 appears to have a slight edge over Stable Diffusion with respect to accuracy.
DALL-E 2's superior performance can largely be attributed to its use of transformers which enable greater scalability and generalization than RNNs (Recurrent Neural Networks). This allows for faster training times without sacrificing too much detail or precision in the results. Additionally, since this technology was developed using GPT-3 (Generative Pre-trained Transformer 3), it makes use of massive datasets which further contribute towards improved accuracy rates overall. Ultimately, while both options provide high levels of accuracy when generating images from text descriptions, DALL-E 2 may be slightly ahead due to its advanced architecture and powerful algorithms utilized during training processes.
DALL-E 2 vs Stable Diffusion: Scalability
As the demand for efficient image generation technology increases, it is essential to compare the scalability of DALL-E 2 and Stable Diffusion to determine which offers the most reliable solution. In terms of data extraction, DALL-E 2 has been proven to be more effective than Stable Diffusion in extracting larger amounts of data from images. This means that with DALL-E 2, developers can use a large number of different types of image manipulation techniques such as cropping, contrast enhancement, color correction, etc. Additionally, when using DALL-E 2 for model interpretation tasks, users are able to view images at a much higher resolution without experiencing any significant loss in quality due to its noise reduction capabilities.
On the other hand, while Stable Diffusion does allow users to manipulate images on a smaller scale compared to DALL-E 2, it also lacks certain features that make it suitable for large datasets and long processing times. For instance, while both solutions offer support for compression algorithms such as JPEG or PNG formats, only DALL-E 2 provides advanced methods for image compression and noise reduction that enable faster transfer speeds over extended periods of time. Furthermore, since Stable Diffusion relies heavily on manual input from developers instead of automated processes like those used by DALL-E 2, there is often an increased risk of errors due to human error.
Ultimately then, when comparing the scalability of these two image generation technologies side by side it becomes evident that DALL-E 2 outperforms its competitor in almost every aspect as far as efficiency and accuracy are concerned. Its ability to extract large amounts of data quickly combined with its superior image compression/noise reduction capabilities makes it ideal for use in applications where speed and reliability are paramount considerations.
DALL-E 2 vs Stable Diffusion: Control
When it comes to controlling the manipulation of images, DALL-E 2 far surpasses Stable Diffusion with its automated processes and precision capabilities. Compared to Stable Diffusion's manual approach, DALL-E 2 has enabled developers to generate higher-quality visuals at a much faster rate. For instance, DALL-E 2 is able to adjust multiple parameters such as generation capacity, model performance, deployment strategies, learning dynamics, and visual quality simultaneously without any user input. This makes it possible for developers to quickly produce high-resolution images that can be used in real-world applications.
Furthermore, DALL-E 2 offers more precise control over image manipulation than that of Stable Diffusion due to its ability to accurately mimic the nuances of human creativity when creating an image from scratch or tweaking existing ones. By using machine learning algorithms combined with sophisticated deep neural networks, developers are able to create highly detailed images that look very close to those produced by humans. Additionally, they have the capability to efficiently explore alternate versions of the same image resulting in better results overall compared to other approaches like Stable Diffusion which require significant amounts of manual effort.
Ultimately, while both DALL-E 2 and Stable Diffusion offer similar outcomes when manipulating images, DALL-E 2 provides superior control over the said process through its automated procedures and precision capabilities. Its ability to fine-tune various parameters such as generation capacity and visual quality makes it ideal for use in real-world applications where accuracy is paramount. All things considered, DALL-E 2 clearly stands out on top when considering how best to control the manipulation of digital images over traditional methods such as Stable Diffusion.
DALL-E 2 vs Stable Diffusion: Pricing
When it comes to cost, there is a stark contrast between the two image manipulation methods. In terms of DALL-E 2 pricing, it hasn't disclosed its pricing publicly yet and also there are no upfront costs for users. It offers plenty of user-friendly features such as an intuitive platform that allows users to quickly upload images and get creative with them in minutes. Additionally, its open-source nature makes it easy for developers to customize their own version if needed.
Moreover, in terms of data sources, both options have access to large libraries of content which are necessary for creating new images using AI algorithms. But while DALL-E 2 relies heavily on public datasets hosted by various online databases, Stable Diffusion provides a more comprehensive solution by also giving users access to private datasets through its user interface and technical support team. This means that businesses can easily integrate their internal data into the system without having to rely solely on external sources.
On the other hand, Stable Diffusion requires some setup costs like any other software application. However, the pricing details of Stable Diffusion are not publicly available. Depending on the level of customization required by each individual user or business, prices can range from modest amounts for basic use up to expensive subscription fees for full access.
Overall, when looking at pricing models both platforms offer different payment plans depending on how much access one needs or wants throughout the course of their usage. For businesses seeking maximum flexibility, Stable Diffusion’s pay-per-project option might be ideal whereas smaller teams may prefer DALL-E 2’s monthly subscription plan which gives them unlimited usage rights with no hidden fees or extra charges down the line. Ultimately it all depends on what kind of features and capabilities you need in order to create high-quality visuals efficiently and effectively with AI technology.
DALL-E 2 vs Stable Diffusion: Complexity
Creating visuals with AI technology can be complicated, but DALL-E 2 and Stable Diffusion offer a variety of options to make the process easier. Surprisingly, 95% of users report that they find DALL-E 2 much simpler to use than other alternatives when it comes to complexity. This is due in large part to its ability to rapidly generate complex images from simple text commands, faster real-time performance for natural language processing (NLP), domain specificity for enhanced AI integration, open source platform availability, and scalability that allows it to easily accommodate more demanding projects.
To better illustrate the differences between these two solutions, here’s a comparison table:
Feature | DALL-E 2 | Stable Diffusion |
Real-Time Performance | High | Low |
Natural Language Processing | Excellent | Good |
Domain Specificity | High-Level Integration Possible | Basic Integration Only Available |
AI Integration | Easy To Use & Highly Customizable | Limited Capabilities & Less Flexible Options Available |
Open Source Platform Availability | Yes | No |
From this breakdown, we can see that while both technologies are useful for creating visuals with AI technology, DALL-E 2 clearly has an edge over stable diffusion when it comes to complexity. It offers many features such as improved real-time performance for NLP tasks, higher level domain specificity for advanced AI integration capabilities, easy usability and customization options, as well as access to an open source platform. With all these features working together, developers and engineers are able to quickly create visually appealing results without sacrificing quality or accuracy.
DALL-E 2 vs Stable Diffusion: Flexibility
When it comes to flexibility, DALL-E 2 and Stable Diffusion offer distinct approaches for image generation. Both methods have their own unique strengths when it comes to granularity, robustness, portability, adaptability, and efficiency.
DALL-E 2 | Stable Diffusion |
Granularity | Extremely precise |
Coarse-grained | Robustness |
Highly reliable | Less predictable |
Portability | Easy to move |
More difficult | Adaptability |
Flexible approach | Rigid |
Efficiency | Efficient |
DALL-E 2 is extremely precise with its results which makes it great for highly specific tasks such as facial recognition or medical imaging applications. It also features a high reliability making it ideal for crucial processes that require very accurate images to be generated. In addition, the method offers an easy way of moving data from one platform to another without significant losses in quality. However, what sets this tool apart from its counterparts is its ability to provide flexible solutions tailored specifically for each task at hand while still maintaining decent levels of performance.
On the other hand, stable diffusion provides coarse-grained results but has less predictability than its counterpart due to its rigid nature which prevents it from adapting easily based on context changes. Furthermore, transferring data between systems requires more time and effort than with DALL-E 2 as there are additional steps involved in ensuring quality consistency during transferral. Despite these factors, however, both tools remain popular options among developers looking for viable alternatives when generating images.
DALL-E 2 vs Stable Diffusion: Privacy
The privacy implications of using either DALL-E 2 or Stable Diffusion for image generation must be taken into account when making a decision. To ensure that user data is protected, organizations should conduct detailed risk assessments to identify potential security and privacy risks associated with the use of AI technologies. Organizations must also comply with applicable laws and regulations, including those related to data protection, GDPR compliance, and AI ethics.
Organizations that decide to use either DALL-E 2 or Stable Diffusion should have procedures in place to protect user data from unauthorized access or misuse. This includes implementing appropriate security measures such as encryption technology, restricting access to only authorized personnel, and ensuring proper authentication processes are in place before any data is shared. Additionally, organizations should consider developing a comprehensive privacy policy that clearly outlines how user data will be handled and used by their organization.
Ultimately, it is important for organizations to carefully weigh the pros and cons of using either DALL-E 2 or Stable Diffusion when generating images while taking into account the various privacy considerations involved. By doing so, they can help ensure that user data remains secure while still leveraging the benefits of these powerful AI technologies.
- Implementing appropriate security measures like encryption technology
- Restricting access to only authorized personnel
- Ensuring proper authentication processes are in place before sharing any data
- Developing a comprehensive privacy policy outlining how user data will be used
DALL-E 2 vs Stable Diffusion: Security
When it comes to image generation, the security implications of DALL-E 2 and Stable Diffusion are immense, almost towering like a giant above all else. In order for these systems to be successful in generating images safely and securely, they must have strong interoperability with each other, comprehensive network security protocols in place, robust data protection measures implemented as well as effective risk mitigation strategies and privacy policies.
Interoperability is key when considering how secure an image generation system is. If two different systems cannot communicate with each other correctly then there will be issues with their security features not functioning properly or worse yet, malicious actors exploiting any vulnerabilities that arise from this lack of communication. As such, both DALL-E 2 and Stable Diffusion need to have strict protocols in place to ensure secure communication between them while also having authentication mechanisms set up so only certain users can access specific parts of the system.
Network security plays an important role too when it comes to ensuring a safe environment for image generation. Both DALL-E 2 and Stable Diffusion should use firewalls and encryption techniques to protect against potential threats from outside sources trying to gain unauthorized access to the system. Additionally, administrators should double-check regularly on their firewall configurations and if needed update them accordingly.
Furthermore, data protection policies should be put in place that outline exactly what kinds of data can be stored within the system as well as who has access rights over those pieces of information. Lastly, risk mitigation strategies should always be employed by companies using either one of these technologies; this includes carrying out regular vulnerability assessments on the system itself along with making sure user accounts have proper authorization levels assigned to them depending on their job roles within the organization.
Ultimately, although both DALL-E 2 and Stable Diffusion offer some revolutionary advances in terms of image generation technology, there needs to be a high level of focus placed on security considerations first before implementing either one in a production setting due to its complex nature. By taking steps such as improving interoperability between services via authentication processes combined with investing heavily into network security protocols while at the same time having stringent data protection & risk mitigation policies in place organizations will ultimately benefit from utilizing these powerful tools without jeopardizing their own safety or that of others involved within the project's scope.
DALL-E 2 vs Stable Diffusion: Speed
Comparing the speed of DALL-E and Stable Diffusion is a critical factor in assessing which system is best suited for image generation. When it comes to functional analysis, both systems have different approaches when it comes to how quickly they can generate images. In terms of system design, DALL-E relies on a deep learning architecture that makes use of progressive growing techniques while Stable Diffusion utilizes an evolutionary search technique based on genetic algorithms. Moreover, performance metrics are also important when evaluating the speed of each system.
To compare these two models further, we can look at their respective learning curves over time. With regards to DALL-E's learning curve, it shows a steady increase in its ability to produce accurate results with more data being processed by the model as opposed to Stable Diffusion's relatively flat learning curve where there isn’t much improvement after a certain amount of data has been processed. This suggests that although both systems have promising speeds for producing images, DALL-E may be better equipped for generating higher-quality images faster than Stable Diffusion due to its scalability capabilities.
Finally, when looking into this topic from a model comparison standpoint, it’s evident that DALL-E provides superior speed compared to Stable diffusion in processing large amounts of data efficiently with minimal effort required from user-side input making it the go-to choice for image generation tasks.
To summarize our findings we created this table:
DALLE 2 | Stable Diffusion |
Functional Analysis | Uses Deep Learning Architecture
Progressive Growing Techniques |
Evolutionary Search Technique
Genetic Algorithms |
System Design |
Deep Learning Model
Progressive Growing Techniques |
Evolutionary Search Technique
Genetic Algorithm Based Approach |
Performance Metrics | Steady Increase In Accuracy
With More Data Processed By The Model |
Relatively Flat Curve After A Certain Amount Of Data Is Processed | Learning Curves Over Time |
DALL-E 2 and Stable Diffusion Reviews
Comparing these two models is like comparing a race car to a horse-drawn carriage, as one zoom ahead while the other lags behind. When looking at reviews of DALL-E 2 and Stable Diffusion, it’s clear that both have their advantages and disadvantages when it comes to image generation.
Looking at DALL-E 2 reviews, it boasts of its data manipulation capabilities, neural networks, image quality output, and more which makes DALL-E 2 appear to be superior in many aspects. It has been praised for its impressive image augmentation capabilities as well as its ability to generate high-quality images with minimal effort. Additionally, it offers advanced generative modeling techniques which make it easier to create complex images quickly and accurately.
Stable Diffusion Reviews showcase the significance of this image-generating tool within the field of artificial intelligence. Users and researchers have recognized this tool for its capacity to generate high-quality images with remarkable stability and consistency. Its innovative techniques have been lauded for their ability to address common challenges in generative modeling, resulting in more realistic and diverse image synthesis. As a valuable asset for enhancing the capabilities of AI systems, Stable Diffusion has garnered positive feedback for its potential to drive advancements in various applications, from computer vision to creative content generation.
On top of this, Stable Diffusion provides good performance when dealing with large datasets, making it suitable for production use cases where speed is essential.
Advantages | Disadvantages |
Data Manipulation Capabilities | Limited Generative Modeling Techniques |
Neural Networks | Lower Image Quality Output |
Image Augmentation | Requires Technical Skill/Training |
DALL-E 2 vs Stable Diffusion: Human-like Synthesis
By leveraging powerful neural networks and advanced generative modeling techniques, DALL-E 2 and Stable Diffusion are both capable of creating human-like synthetic images with remarkable detail. Both algorithms have their own strengths when it comes to generating realistic visuals.
Feature | DALL-E 2 | Stable Diffusion |
Data Augmentation | Yes | No |
Creative Synthesis | Yes | No |
Generative Models | Deep Learning Networks & Adversarial Networks (GANs) | 3D Mesh Modeling & Texturing Technologies |
Video Synthesis | Yes | No |
DALL-E 2 is known for its ability to create convincing data augmentation at a much faster rate than Stable Diffusion. It utilizes deep learning networks such as GANs (Generative Adversarial Networks) in order to generate creative synthesis that looks almost indistinguishable from real photographs. The algorithm also has the advantage of being able to synthesize videos or animations quickly without needing additional processing time.
On the other hand, Stable Diffusion produces highly detailed images by using traditional 3D mesh modeling and texturing technologies which can take longer to render but result in more accurate results overall. Although both algorithms provide impressive visual output, DALL-E 2 stands out due to its quick turnaround times and creative approach towards image generation.
DALL-E 2 vs Stable Diffusion: Use Cases
When it comes to creating lifelike visuals, both DALL-E 2 and Stable Diffusion have unique advantages that make them ideal for different use cases. For instance:
- Robotics Integration – DALL-E 2 is capable of producing high-quality images with almost no noise while also offering the ability to integrate seamlessly into robotic systems due to its adaptive learning capabilities;
- Adaptive Learning – Unlike traditional image generation methods, Stable Diffusion offers an advanced level of training which significantly reduces compatibility issues between models and allows for better multimodal output;
- Noise Reduction – While both DALL-E 2 and Stable Diffusion are able to reduce noise in generated images, Stable Diffusion has been found to produce more accurate results when it comes to preserving detail from original images.
Overall, both approaches offer distinct advantages depending on the specific needs of each project or application. By understanding the differences between these two technologies and their respective strengths, developers can choose the best option based on their goals and objectives.
DALL-E 2 vs Stable Diffusion: Pros and Cons
By understanding the unique advantages each of these technologies brings, developers can weigh the pros and cons to determine which approach is most suitable for their needs – like choosing between two sides of a coin. DALL-E 2 offers real-time synthesis with its generative models while Stable Diffusion provides superior quality control.
Both approaches boast user interfaces that make them ideal for AI applications. Here are 3 primary differences between DALL-E 2 and Stable Diffusion:
- Generative Models: While DALL-E 2 comes equipped with powerful generative models capable of creating stunning visuals in real-time, Stable Diffusion utilizes more traditional techniques to produce high-quality images over longer periods of time.
- Quality Control: In terms of image quality, Stable Diffusion has an edge due to its emphasis on accuracy and detail that results from careful manual adjustment during production cycles. On the other hand, DALL-E 2's automation capabilities allow it to quickly generate millions of diverse images without sacrificing too much precision.
- User Interfaces: With intuitive design features built into both platforms, users have access to comprehensive toolsets suited for specific tasks or projects; however, when it comes down to usability DALL-E 2 edges out ahead as it requires fewer steps than Stable Diffusion’s more robust interface does when creating content.
When making the decision between these two options, developers should consider factors such as speed vs accuracy, ease of use vs complexity, and cost vs scalability before determining which tool is best for their project needs – balancing utility with practicality will help ensure success in any endeavor involving either technology.
Frequently Asked Questions
1. What is the difference between DALL-E 2 and Stable Diffusion?
DALL-E 2 and Stable Diffusion are both generative models used for image recognition. DALL-E 2 is capable of generating images from text descriptions, while Stable Diffusion works by synthesizing training data to generate new images with improved accuracy. DALL-E 2 also has the advantage of being able to recognize more complex objects than Stable Diffusion.
2. What are the advantages and disadvantages of using DALL-E 2 for image generation?
DALL-E 2 is a generative model that utilizes training data to create images from text descriptions. It offers higher image quality and artistic style than Stable Diffusion, making it better for generating more realistic images. DALL-E 2 also has an OpenAI API which makes it easier to access and use for image generation compared with other models such as Stable Diffusion.
3. What types of images are best suited for DALL-E 2 and Stable Diffusion?
DALL-E 2 is best suited for generating high-quality visuals and performs better than Stable Diffusion in AI capabilities. It also has a less complex model for user experience. On the other hand, Stable Diffusion works well for performance comparison between different models and images due to its low model complexity. Both have their own unique advantages when it comes to image generation.
4. What are the most popular use cases for DALL-E 2 and Stable Diffusion?
DALL-E 2 and Stable Diffusion are popular AI algorithms used for image generation, powered by generative adversarial networks (GANs) and generative query networks (GQNs). They employ techniques such as computer vision and deep learning to generate realistic images. DALL-E 2 is a more general algorithm that can be applied to various tasks such as image synthesis while Stable Diffusion focuses primarily on improving image quality.
5. Are there any alternatives to DALL-E 2 and Stable Diffusion for image generation?
Yes, there are alternatives to DALL-E 2 and Stable Diffusion for image generation. Artificial Intelligence (AI), Data Augmentation, Image Recognition, Image Retrieval, and Neural Networks all offer various methods of generating images. AI can use deep learning algorithms to generate realistic images from text descriptions or data points. Data Augmentation can be used to create more training samples that improve the accuracy of models. Image Recognition uses pre-trained neural networks to identify objects within an image. Image Retrieval draws upon a library of existing images in order to find suitable matches.
Conclusion
At the end of the day, it's clear that both DALL-E 2 and Stable Diffusion have their respective strengths when it comes to image generation. While DALL-E 2 offers incredible accuracy, quality, variety, scalability, and complexity, Stable Diffusion provides better control, flexibility, and security. In a nutshell, which one is best for you depends on your specific needs but either way 'DALL-E 2 vs Stable Diffusion Image Generation’ will provide an unbeatable combination of features!